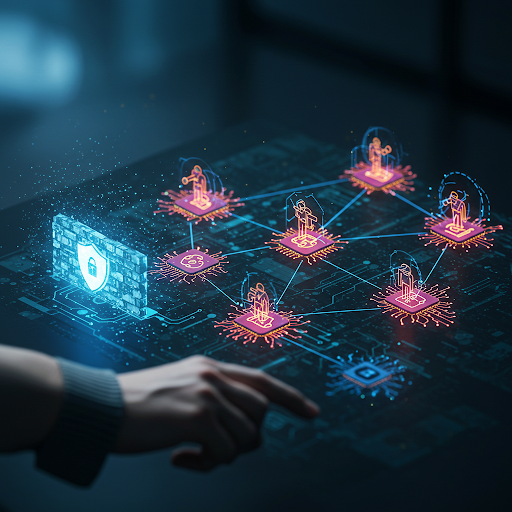
Gartner predicts that by 2027, 17% of cyberattacks will involve Generative AI.
As artificial intelligence (AI) technologies continue to advance, they are rapidly becoming essential across various sectors in the UK, including healthcare, finance, manufacturing, and education. AI’s ability to analyse vast datasets and automate processes enhances operational efficiency and delivers significant competitive advantages, allowing businesses to make data-driven decisions with incredible speed and accuracy. For example, AI is used in healthcare to improve diagnostic accuracy, personalise treatment plans, and streamline administrative workflows, ultimately leading to better patient outcomes.
However, the journey of AI adoption is not without challenges, particularly concerning security and ethical considerations. Integrating AI systems can expose organisations to cybersecurity risks, including data breaches and misuse of sensitive information. Additionally, ethical concerns surrounding decision-making transparency and bias in AI models necessitate robust governance frameworks. Strong governance frameworks are crucial in defining clear guidelines and policies to manage these cyber risks effectively. Such frameworks enhance security and encourage innovation by providing safeguards to build stakeholders’ trust. Organisations can foster an environment that balances innovation with ethical considerations by prioritising responsible AI use, ultimately leading to more secure and efficient adoption of AI technologies across the UK.
The Importance of Safeguarding AI Systems
Protecting AI systems and your company’s precious data is paramount in our rapidly evolving technology landscape. As cybersecurity threats grow in sophistication, they threaten data integrity and can lead to breaches that jeopardise an organisation’s reputation and financial well-being. Thus, businesses are called to create a robust security framework that safeguards their AI infrastructure and paves the way for effective and transparent adoption.
Key Cyber Security Challenges
Before embarking on implementing a security framework tailored for AI technologies, it is crucial to thoroughly understand the multitude of challenges that businesses encounter during this integration. Here are some key considerations:
1. Evolving Threat Landscape: The environment in which AI operates is continuously changing, and AI systems are particularly vulnerable to various sophisticated threats. These include adversarial attacks, where malicious inputs can deceive the AI algorithms; data poisoning that compromises the training data; and model theft, where competitors or hackers replicate an organisation’s proprietary models. As a result, organisations need to adopt specialised security measures tailored to defend against these unique risks.
2. Complexity of AI Systems: The intricate nature of AI models and their myriad dependencies complicates the process of securing them. Businesses must deeply understand the AI systems, infrastructure, and data pipelines supporting them. This challenge is especially pronounced for organisations with limited expertise in AI, making it essential to seek out specialists or invest in training to navigate and secure these complex systems effectively.
3. Resource Constraints: Many businesses in the UK, especially small to medium-sized enterprises (SMEs), often operate under strict budget constraints. This financial limitation can hinder their ability to deploy comprehensive cybersecurity measures or hire dedicated personnel. However, creative solutions, such as using open-source security tools, outsourcing security tasks, or investing in employee training, can help bolster their defences within their budgetary constraints.
4. Data Security: AI systems typically require access to vast quantities of data, which can be highly sensitive (e.g., personal information and financial records). Exposing this data to unauthorised access or exploitation can have serious ramifications, including reputational harm and legal penalties. As such, organisations must implement robust data security practices, including strong encryption protocols and stringent access control measures, to protect sensitive information.
5. Model Security: The AI models can be prime targets for adversarial attacks, which seek to manipulate the algorithms to produce erroneous outputs. To counter this, organisations must employ ‘adversarial training’, which involves training the AI model with adversarial examples to make it more robust. Continuous monitoring, on the other hand, involves regularly checking the AI system for any suspicious activity or potential threats and maintaining detailed logs for incident response.
6. Compliance and Ethical Use: organisations must navigate a complex landscape of regulations regarding data protection and ethical AI usage, such as the General Data Protection Regulation (GDPR). Non-compliance with these regulations can result in severe legal consequences, including hefty fines and damage to reputation. Establishing a clear framework for compliance and ethical considerations is imperative for responsible AI deployment.
7. Supply Chain Risks: AI technologies often rely on external third-party services and vendors. If these suppliers do not implement robust security practices, they can introduce significant vulnerabilities into an organisation’s operations. Performing due diligence on these partners is essential, ensuring they adhere to stringent security standards to safeguard the entire supply chain.
8. Employee Training and Awareness: Many security vulnerabilities arise from human error. To mitigate these risks effectively, employees must have the knowledge and skills to adhere to AI security protocols. Regular training sessions, awareness campaigns, and a culture of security mindfulness can significantly decrease the likelihood of human error leading to security breaches.
Businesses can create a more resilient security posture businesses can create a more resilient security posture by proactively addressing these challenges.
Strategies Used to Secure AI Models
- Leverage Open-Source Tools and Frameworks: To implement security features, use open-source libraries like TensorFlow Privacy and Adversarial Robustness Toolbox (ART). Explore free or low-cost vulnerability scanning and penetration testing tools for AI systems.
- Adopt Cloud-Based Security Solutions: For AI services, use cloud providers’ built-in security features, such as data encryption, access control, and threat detection. Consider cloud-based security platforms that offer AI-specific security tools and services on a subscription basis.
- Implement Security Best Practices: Data Security: Ensure data used for training and inference is secure, anonymised, and protected against unauthorised access.
- Model Security: Implement measures to protect AI models from theft, manipulation, or unauthorised access.
- Access Control: Enforce strict access control policies for AI systems, limiting access to authorised personnel only.
- Continuous monitoring and logging are essential components of a robust security framework. By vigilantly monitoring AI systems, organisations can detect and respond to suspicious activity promptly, enhancing their security posture and instilling a sense of control.
- Prioritise Risk-Based Approach: Identify critical AI applications and prioritise security measures based on their potential impact and vulnerability. Focus on first addressing the most significant risks to maximise security within budget constraints.
- Invest in Training and Awareness: Train employees on AI security best practices and potential threats. Raise awareness about the importance of secure AI development and deployment.
- Participating in industry forums and communities to share knowledge and best practices on AI security is a powerful way to stay informed and connected. By leveraging government resources and initiatives, such as the National Cyber Security Centre (NCSC), for guidance and support, organisations can strengthen their security efforts and feel supported in their AI adoption journey.
- Consider Managed Security Services: For businesses with limited in-house expertise, consider outsourcing AI security management to specialised providers. Managed security services can offer cost-effective monitoring, threat detection, and incident response solutions.
Considerations When Designing a Robust Security Framework
1. Conduct a Risk Assessment
The foundation of a solid security framework is an exhaustive risk assessment. This initial step involves identifying all assets and data points associated with AI systems, evaluating potential vulnerabilities, and prioritising these risks based on their impact and likelihood. Key focus areas include:
– Data Storage and Access: Assess where your AI-related data is stored and who has access.
– Network Security: Evaluate the network’s security connecting your AI systems.
– Compliance: Ensure compliance with all relevant regulations.
2. Establish Security Policies and Procedures
Once risks are identified, establish comprehensive security policies that address these vulnerabilities. These policies should govern how data is handled, processed, and stored. Essential elements include:
– Data Encryption: Implement encryption for sensitive data at rest and in transit.
– Access Control: Utilize role-based access controls to limit data access to authorised personnel only.
– Incident Response Plan: Develop a clear response plan for security breaches and incidents.
3. Design Secure AI Systems
Security considerations should be integrated into the design phase of AI systems. This includes:
– Robust Authentication Protocols: Implement multi-factor authentication processes for access to AI systems.
– Secure Model Development: Employ secure coding practices while developing AI algorithms and regularly test for vulnerabilities.
4. Data Privacy and Governance
Implement strict data governance policies to manage data collection, sharing, and processing. Focus on:
– Data Minimization: Only collect the data necessary for your AI models.
– Data Anonymization: Where applicable, anonymise data to enhance privacy while allowing for valuable AI insights.
– Compliance: Ensure compliance with GDPR and other pertinent data protection laws.
5. Continuous Monitoring and Threat Detection
Leverage AI technologies themselves to enhance cybersecurity through continuous monitoring systems. These systems can proactively identify unusual patterns or potential breaches:
– Anomaly Detection: Utilize AI algorithms to monitor user behaviour and flag anomalies.
– Regular Audits: Conduct security audits of your AI systems to identify and mitigate risks.
6. Training and Awareness Programs
Develop training programs catering to all employees, emphasising AI security best practices. Key components should include:
– Phishing Awareness: Equip employees to recognise and react to phishing attempts.
– Data Handling Procedures: Instruct staff on effective data management practices.
7. Collaboration and Knowledge Sharing
As AI security continues to evolve, collaborating with industry peers can enhance your security practices. Engaging in security forums and initiatives fosters the sharing of strategies and experiences that can lead to better outcomes.
Security Framework Implementation Roadmap Based On ISO 42001 (AI Management System)
This roadmap provides a structured timeline for implementing ISO 42001, the gold standard international AI Management System, ensuring key milestones are met with appropriate cost estimates. Adjustments can be made based on specific organisational needs and resources.
Conclusion
As UK businesses rapidly embrace AI technologies, establishing a robust security framework isn’t just important; it’s essential. The risk of data breaches and cyber threats is ever-present, and the potential consequences can be devastating. To protect against these vulnerabilities, organisations must proactively understand security challenges, implement comprehensive policies, and foster a culture of awareness and collaboration. By doing so, they safeguard their AI systems and unlock their full potential, fueling innovative growth and securing a competitive advantage in the market. Investing in cost-effective cybersecurity measures for AI applications is a smart, strategic move that calls for a proactive, risk-based mindset. UK businesses can bolster their AI security without breaking the bank by leveraging open-source tools, cloud-based solutions, and industry best practices.
The key lies in continuous learning and adaptability; staying informed about evolving threats is crucial for ensuring the long-term security of AI deployments. Now is the time for UK businesses to take decisive action. By prioritising AI security, they can protect their valuable data and pave the way for transformative advancements that drive success and sustainability in an increasingly digital landscape. Secure your AI systems today and position your organisation for enduring growth and resilience in the face of future challenges. Don’t wait; act now to safeguard your AI journey!